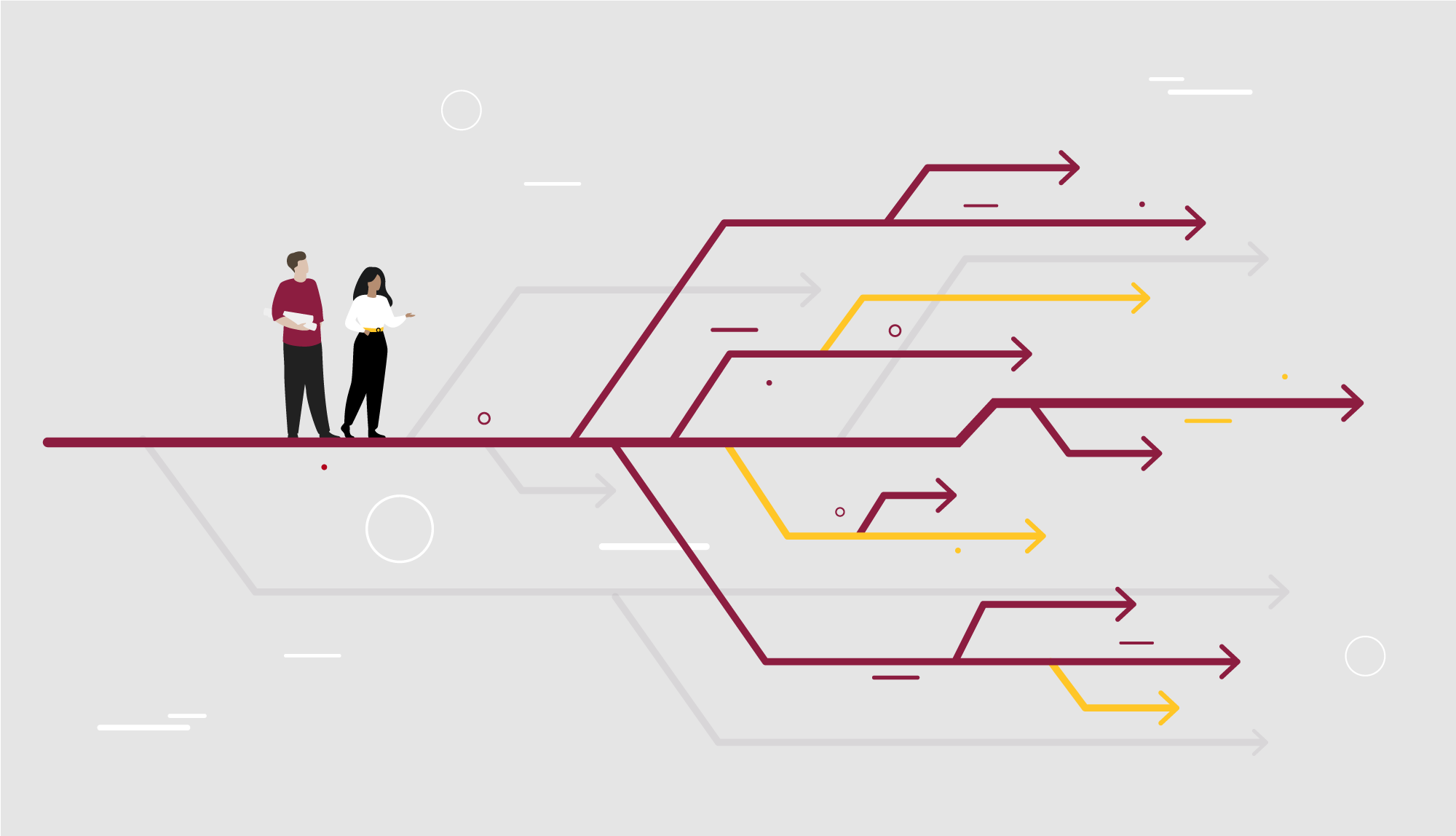
>> MS-BA future students
MS-BA big data track
Combining the latest developments in industry with a strong foundation in research and cross-disciplinary application, the ASU MS in business analytics with a big data track will deepen your quantitative and analytical skills, and you’ll discover how to derive value from data and modeling, lead data-driven analyses, and create a business advantage across markets and industries.
At a glance
9-month program
30 credit hours
Top hiring companies
Career paths and outcomes
$131,490
Median salary for computer information and research scientists
with a master’s degree
Bureau of Labor Statistics, 2022
22%
Job growth | 2020 to 2030
Much faster than average for all careers
Bureau of Labor Statistics, 2022
A career in cloud computing or tech consulting offers both a variety of unique pathways, but a rewarding salary — especially for those with a master’s degree. Sample job titles include:
- Business Intelligence Analyst
- Data Scientist
- Data Analyst
- Data Engineer
- Data Architect
- Machine Learning Scientist
- Machine Learning Engineer
- Business Intelligence Developer
Big data course descriptions
Ensuring the foundational understanding of contextualized analytics within the business enterprise continuum by covering how data flows and is managed across the landscape of enterprise business processes.
Provides a foundation in programming fundamentals, the skills to combine and manipulate structured and unstructured data, and the ability to summarize, visualize, and draw insights from the data. This course may be waived with demonstrated Python proficiency.
Provides a solid foundation and deeper understanding of the use of quantitative modeling tools and techniques to solve problems faced in modern supply chains. Uses Excel workbooks to implement the appropriate quantitative methods, including forecasting demand, capacity planning of a manufacturing line and the line cycle time as it pertains to parts inventory management.
Charting a roadmap for data-driven decision making and getting a practical understanding of how IT tools and techniques can allow managers to extract predictive analytics and patterns from primarily numeric data.
Addresses the use of analytics tools and techniques to enhance the ability of quality management approaches to improve processes. Introduces modern quality management approaches including six sigma and design for six sigma. Covers the define, measure, analyze, improve and control (DMAIC) improvement cycle: the core process used to drive six sigma projects. DMAIC refers to a data-driven improvement cycle used for improving, optimizing and stabilizing business processes and designs. Provides an analytics roadmap to help users work through the DMAIC problem-solving process.
Explains the skills and knowledge necessary for mastery of the use of quantitative modeling tools and techniques to support a variety of business decisions. Also explores deterministic optimization techniques, including linear programming, nonlinear programming, integer programming; network models and a brief introduction to metaheuristics. Covers the use of these models for a variety of common business problems. Practical application of these models uses Excel and standalone software. Also studies how to ensure that these solutions work in a wide variety of situations (what-if analysis).
Explores how to support informed decision making and extract predictive analytics and patterns from nonnumeric data by leveraging tools and techniques to analyze unstructured data.
Addresses the skills and knowledge necessary to model situations where uncertainty is a major factor. Models include decision trees, queuing theory, Monte Carlo simulation, discrete event simulation, and stochastic optimization, along with application for solving a wide variety of common business problems.
Deep learning applications have become an integral part of our lives over the last decade. Alexa, Amazon Go, Waymo, Apple Face ID, and Facebook's face recognition applications are all powered by deep learning networks. Applications based on deep learning models cover a wide spectrum of industries including retail, automotive, manufacturing, health care, banking, insurance, agriculture, security and surveillance. Hands-on look at the latest models, trends and challenges of deep learning applications in business.
Involves formulating critical marketing problems, developing relevant testable hypotheses, collecting and analyzing data and, most importantly, drawing inferences and suggesting actionable implications.
Preparation of a supervised applied project that is a graduation requirement in some professional majors.
MS-BA curriculum
Learn how to discover and implement analytical insights with a leading-edge curriculum from one of the top-ranked business schools in the U.S. With tracks in big data, cloud computing and tech consulting, fintech, marketing analytics, and supply chain analytics, the W. P. Carey MS-BA can prepare you for a wide range of career opportunities.
Explore the MS-BA curriculum and academic tracks